It is now more than necessary to design technology that is more scalable and energy-efficient. However, the inability of conventional computers to compute in an energy-efficient way has led to a search for novel computing methods.
According to Kerem Camsari, an assistant professor of electrical and computer engineering at UC Santa Barbara, probabilistic computers (p-computers) offer the solution. P-computers are powered by probabilistic bits (p-bits), which communicate with one another in the same system. P-bits oscillate between positions and operate at room temperature, in contrast to qubits, which may be in several states at once, or the binary 0 or 1 bits used in conventional computers.


We demonstrated that fundamentally probabilistic computers made of p-bits can outperform cutting-edge software that has been developed over many years, according to Camsari. Along with John Martinis, a professor of physics at UCSB, and Luke Theogarajan, vice chair of the ECE Department, Hisi team collaborated with academics from the University of Messina in Italy. The researchers were able to obtain their encouraging results by using conventional hardware to create domain-specific solutions. They invented the first sparse Ising machine (sIm), a ground-breaking computer system that solves optimization issues with the least amount of energy use.


Camsari claims that the sIm is a group of probabilistic bits that can be compared to people. The “sparse” relationships of the machine are the small number of reliable friends that each individual has. He said, “The individuals don’t have to hear from everyone in a whole network since they each have a limited selection of trustworthy pals and they can make judgments swiftly. “The method by which these agents come to an agreement is comparable to the method used to solve a challenging optimization problem that fulfils several constraints. We can define and address a wide range of these optimization issues using the same hardware thanks to sparse ISing machines.
The team’s prototyped solution made use of a field-programmable gate array (FPGA). With sampling speeds that were five to eighteen times faster than those reached by optimised algorithms on conventional computers, the researchers showed that their sparse design for FPGAs was up to six orders of magnitude faster. They also asserted that their sIm achieves extremely high parallelism, with the number of p-bits scaling linearly with the number of flips per second – the crucial parameter that dictates how quickly a p-computer can reach a rational conclusion.
Camsari refers once more to the example of close friends discussing a choice. The main problem, he said, is that creating a consensus involves robust communication among individuals who frequently converse with one another based on their most recent ideas. “A consensus cannot be achieved and the optimization problem is not solved if everyone takes decisions without listening.”
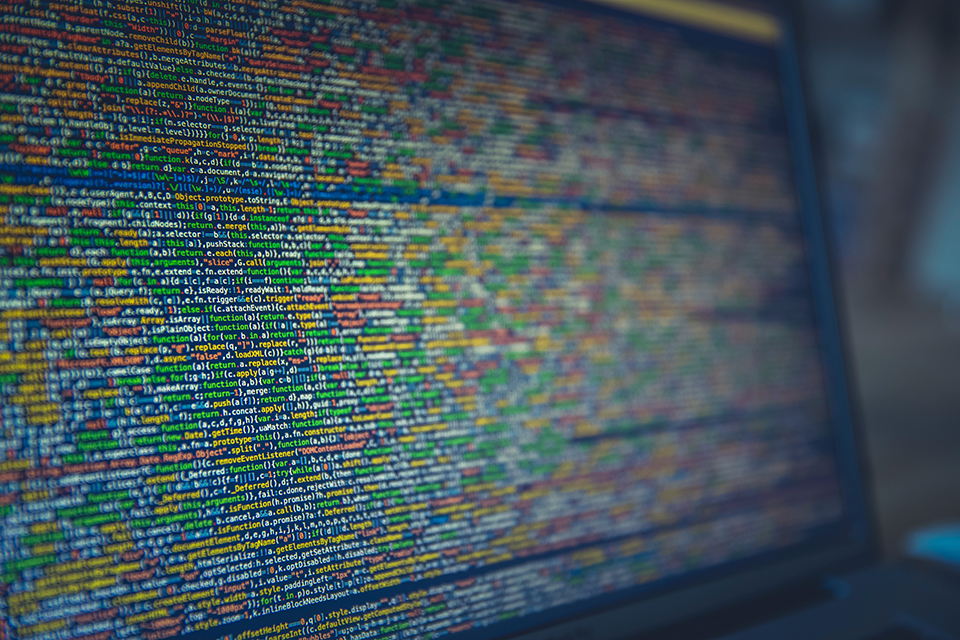
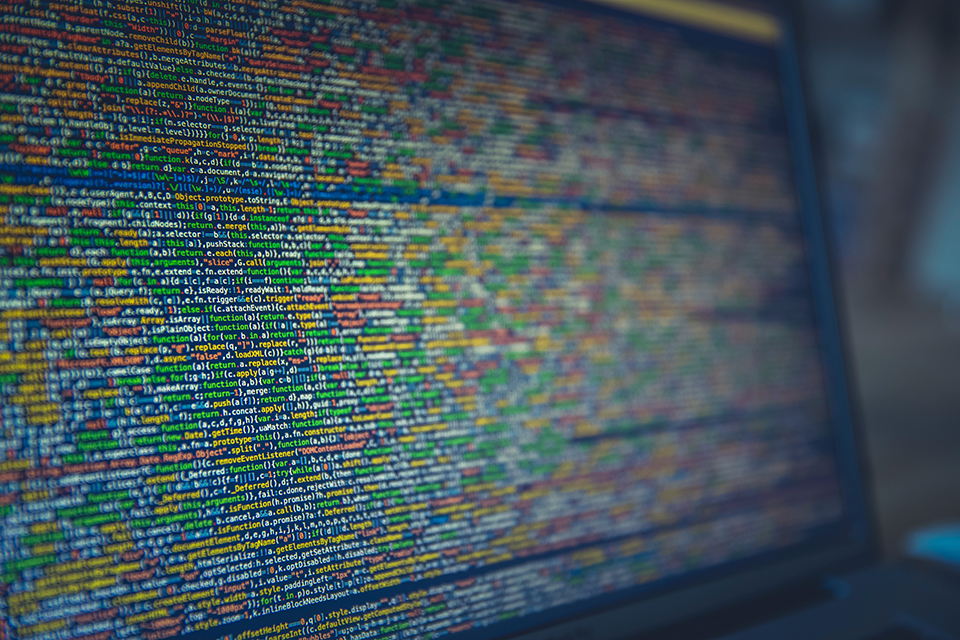
Increasing the flips per second while ensuring that everyone listens to one another is essential because, to put it another way, the faster the p-bits communicate, the faster they can reach a consensus.
“, he clarified. “We parallelized the decision-making process by guaranteeing that everyone listens to each other and restricting the amount of ‘people’ who might be friends with each other.”
He remarked, “To us, these results were only the tip of the iceberg.” “We emulated our probabilistic architectures using existing transistor technology, but the benefits of using nanodevices with considerably greater degrees of integration to develop p-computers would be immense. This is what keeps me up at night.
CLICK HERE TO ACCESS THEIR STUDY