The issue of analogue chips being susceptible to noise or minor fluctuations in voltage that create errors can be accounted for here by learning and conquering, according to ARYABHAT, which uses a learning paradigm. Chetan Thakur, IISc Bangalore professor,
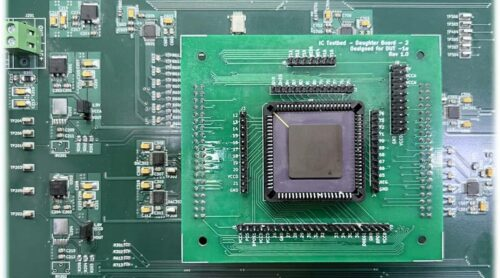
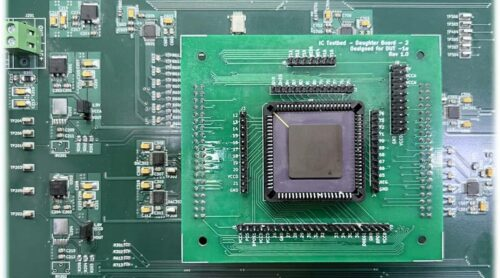
The majority of computing carried out today is digital. However, the environment is analogue, and it serves mostly as the interface. Although the world has greatly benefited from the scalability and precision that digital computation has brought, analogue often serves as the foundation for the digital world. In comparison to analogue chips, digital chips have the advantage of being more easily synthesised and afterwards customised to meet the needs. With only few adjustments, a digital chipset architecture can be used across many technological eras and produces accurate results.
As they are less accurate and even use more power than digital devices, analogue devices have their own unique set of issues. The noise that causes errors is more likely to affect analogue chips. An innovative framework for creating analogue chipsets has been created by a team of researchers at the Indian Institute of Science (IISc), Bangalore. These chipsets could be faster and consume less power than the digital processors found in the majority of gadgets.
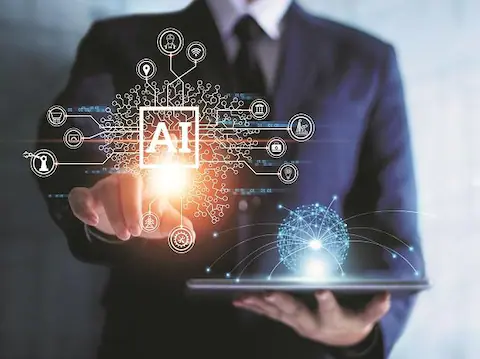
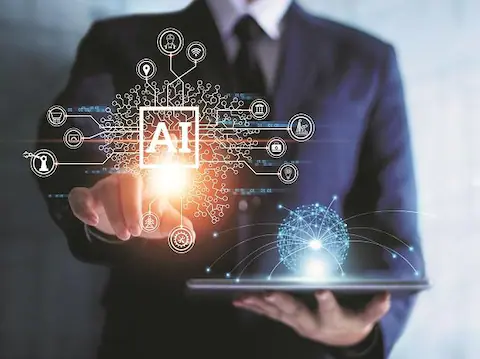
“Analog Reconfigurable Technology And Bias-scalable Hardware for AI Tasks” is the meaning of the acronym ARYABHAT. The chip can be updated and ported to work with many generations of process designs and software. ARYABHAT-1 can quickly process any AI tasks requiring massively parallel computing processes, including speech and object recognition.
“One significant point I would want to make is that the ARYABHAT architecture is ‘bias-scalable,’ meaning that performance is unaffected by changes in operational parameters like voltage or current. This means that the same chipset may be set up for both high-speed operations like object identification and extremely energy-efficient Internet of Things (IoT) applications, Prof. Chetan explains.
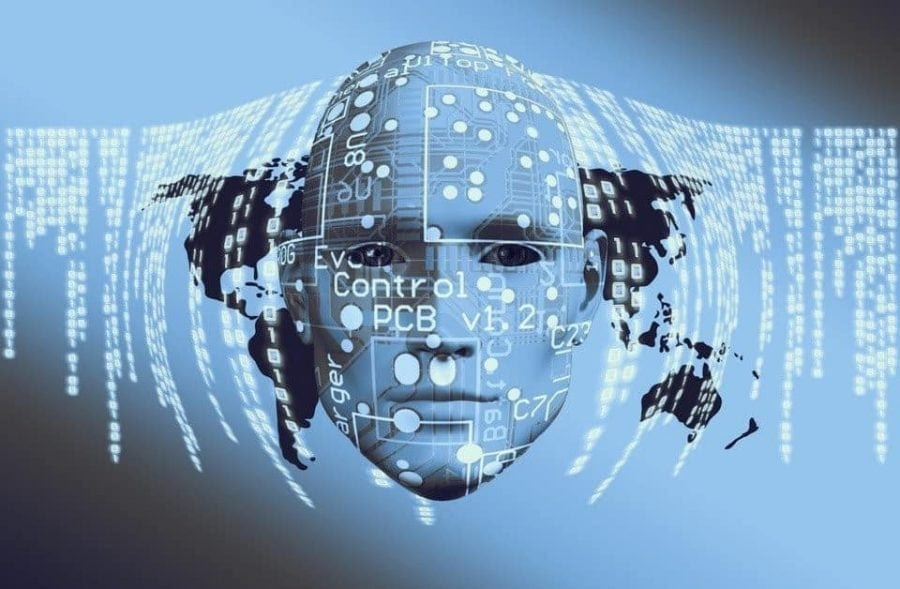
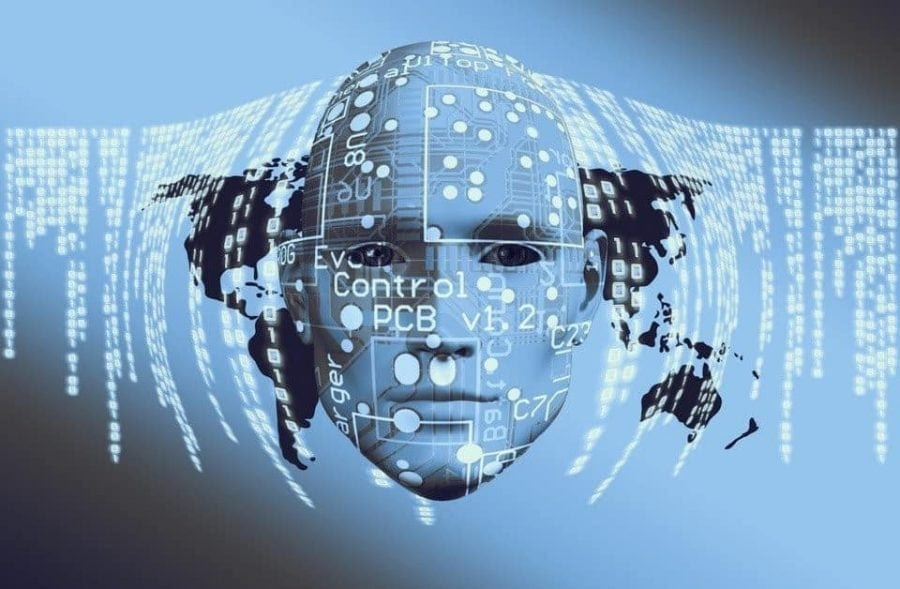
The chip can be configured with different machine learning paradigms and can operate like a digital chip, according to the researchers.